The case for mechanical trading strategies

When it comes to implementing trading strategies, there are many compelling reasons to rely on computers rather than human discretion.
Computers have been used in the investment business for more than 40 years. Initially, fund managers used computers for tasks such as basic math calculations and charting, which at the time was revolutionary. Today, the majority of the daily trading volume in U.S. equities is controlled by highly sophisticated machines, programs, and models making split-second decisions.
Some clients may still feel uncomfortable having their money managed by algorithms instead of human beings, but many others may see the value of mechanical trading strategies that “take emotion out of the equation.”
What are mechanical and discretionary trading strategies?
What do we mean when we say a trading strategy is “mechanical”? It means that all trading actions are determined by a clearly defined set of rules without discretion or room for interpretation. Specifically, a mechanical trading strategy “playbook” covers these three areas:
- Method for selecting instruments available for trading.
- Entry/exit rules (i.e., when to buy or sell and at what price).
- Capital allocation (i.e., how much money to put into each position).
Differences between mechanical and discretionary strategies during their life cycles
The major differences between mechanical and discretionary trading strategies can best be appreciated in the context of a typical trading strategy life cycle (Figure 1), which comprises four fundamental stages:
- Design: Concept development to exploit a market anomaly.
- Validation: Verification of a systematic edge.
- Production: Implementation of live trading.
- Termination: Redesign or abandonment due to deterioration of the market edge.
FIGURE 1: FOUR STAGES OF A TRADING STRATEGY’S LIFE CYCLE
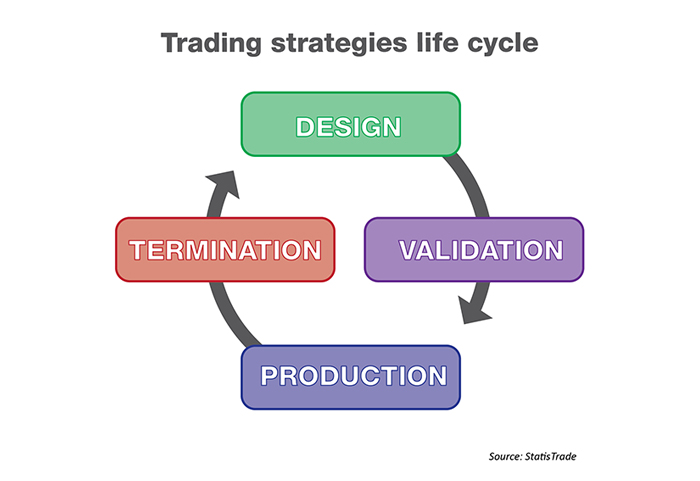
Designing a strategy is a challenging task that typically involves researching historical data to discover an exploitable market edge. Once identified, a process is built that includes trading rules (the “strategy”) that the manager or computer will apply to take advantage of that edge. This stage lays the foundation for success or failure and is prone to many human biases and systematic errors. Preventing them requires rigor and consistency.
Mechanical strategies have the distinct advantage of making the best use of historical data. They allow for a backtesting process that comes as close as possible to simulating the real-life execution of the strategy concept. Without overlooking any trades, a computer can go through large amounts of data in very little time. In contrast, human decisions cannot be consistently reproduced (because of the effects of stress, moods, or subjective judgment) and require significant time to simulate trade results even for short periods.
Validation is the process of making sure that the trading concept provides a real market edge, meaning one likely to persist in the future. In other words, validation focuses on identifying the “fool’s gold” from the real precious metal before getting investors to buy a stake in the prospecting venture.
Discretionary strategies can basically employ only one method of validation: “out-of-sample” (OOS) testing. OOS testing applies the trading concept to data not previously used in the design phase. When performed on historical data, the same problem persists: the inability to consistently simulate human behavior, which makes the validation results questionable. As an alternative, live data can be used (basically building a track record), but this is a slow process that cannot be accelerated.
Of course, these two variations of OOS testing also exist for mechanical trading strategies. But in addition, a method grounded in statistics and derived from the insurance industry called “stochastic modeling” is available.
In short, stochastic modeling applies alterations to the data fed into the strategy and/or to the numerical parameters of its settings, thereby producing many permutations (typically thousands) of simulated performance over a given time period. (For a comprehensive introduction to stochastic modeling, watch StatisTrade partner Dave Walton’s presentation at the 2016 MTA conference or download the accompanying slides.)
This huge collection of virtual outcomes serves as a far better basis for the strategy validation than just a single result (as OOS provides). The quality of the analysis and the confidence an investor can have in it exceeds OOS testing by far. Feedback from our customers has confirmed this advantage. But clients also made us aware of another valuable aspect for them as strategy providers or third-party managers: the potential to distinguish themselves from their competitors. Currently, it’s quite rare to find strategies that were validated with a cutting-edge method like stochastic modeling. Only mechanical strategies offer this option.
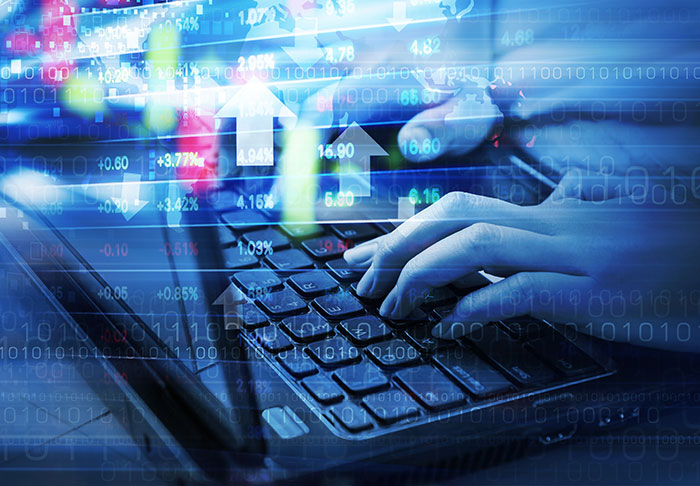
Reaching the production phase is the ultimate goal of strategy development. In this stage, assets are deployed and value creation for clients takes place. The main challenge lies in properly executing the trading concept (e.g., data processing, evaluation, and signal detection) and all individual actions (e.g., capital allocation and order management).
This is another area where mechanical strategies shine, offering the pinnacle of speed, reliability, and quality. Computers clearly outperform humans when it comes to complex calculations and high data volume. Their consistency in applying the concept behind the strategy is unmatched. Flawless execution can be achieved through complete process automation down to the timely placement of all necessary orders. As a result, strategy providers and third-party managers can significantly cut costs and provide unmatched operational excellence to their clients.
Termination is hardly ever talked about when it comes to strategy performance, but it is a critical step. The goal of the termination process is to find out if the strategy is “broken,” or no longer performing as expected. If that’s the case, the strategy may need to be redesigned or even shut down. When the investment process is driven by discretion, it is virtually impossible to say whether the underlying concept has lost its edge, a “normal” streak of bad luck has happened, or if the decision-maker’s abilities are impeded.
On the other hand, mechanical strategies—especially when stochastic modeling was applied in the validation stage—can offer clear-cut numerical thresholds to serve as predetermined trigger points for a reassessment of the strategy. It is even possible to select the degree of certainty (called “confidence level” in statistics) to reflect the different needs of clients with a cautious or more aggressive investment style.
The final case for mechanical trading strategies
Without trying to suggest that computers always outperform investment professionals, it is worth noting that mechanical trading strategies have many unique aspects that can allow them to play an important role in portfolio allocation.
As described, mechanical strategies offer characteristic advantages in all four life-cycle stages (Table 1). When compared to the discretionary approach, the obvious difference is that many of the emotional and behavioral downsides of human decision-making under “live” market pressure are taken out of the active-management process. Yet, in our experience, the most significant benefit typically lies in the possibility of scientifically validating the performance characteristics as part of the due-diligence process. This analysis gives money managers an excellent basis for selecting rule-based active and dynamic strategies that optimally fit their clients’ personal risk profiles.
TABLE 1: ADVANTAGES OF MECHANICAL VS. DISCRETIONARY STRATEGIES
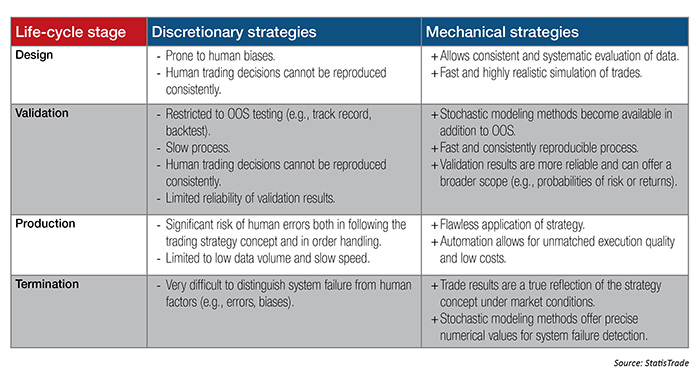
Thomas Krawinkel, MBA&E, was a co-founder and partner at StatisTrade LLC, a trading strategy evaluation firm that worked with fund managers and family offices to evaluate and improve trading system performance using advanced statistical techniques. An engineer by training, Mr. Krawinkel has been active in the markets since the 1980s. He won the National Association of Active Investment Managers (NAAIM) 2011 Wagner Award for insights related to success factors for system performance.